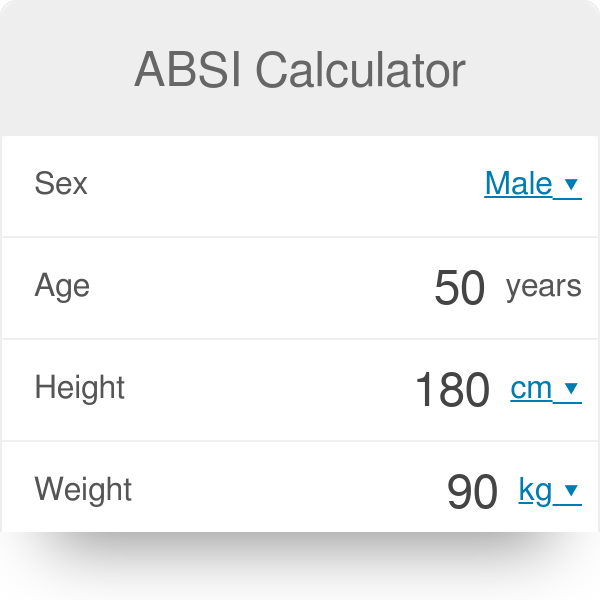
Body shape index -
All analyses were run in duplicate. All variables were checked for normality using the Shapiro-Wilk test. The Pearson correlation coefficients were calculated for logarithmically e-based transformed data. Moreover, biochemical variables were interpreted with respect to lower and upper quartile of BMI and ABSI and the Mann—Whitney test was used for data comparison.
All calculations were carried out using the Statistica v. Baseline characteristics of the participants are presented in Table 1. According to BMI standards Only 2. Circulating glucose and triacylglycerols were higher than normal in similar percentages of students 6.
Slightly more participants 8. On the contrary, higher than normal TC, LDL-C and non-HDL-C were observed in The most important finding of our study concerns ABSI, which is better correlated to changes in circulating TC and insulin than BMI in young sedentary men.
It is worth noting that the homogeneity of our participants according to age and sex strengthens our findings.
On the other hand, it should be stressed that ABSI validity in the prognosis of cardiovascular disease CVD is far from being elucidated since Maessen et al.
It should be stressed that in some way ABSI agrees with the WHO recommendation concerning waist circumference inclusion into health risk evaluation [ 30 ]. Similarly, other authors have suggested that both BMI and WC contribute to the prediction of body adiposity in white men and women [ 38 ].
The importance of WC measurements in diagnosis of health risk has been suggested by many authors since it has been postulated that WC provides indirect information about visceral fat accumulation [ 39 , 40 ].
At present it is well documented that visceral fat due to its location and metabolic characteristics contributes to distorted metabolism to a much greater extent than subcutaneous fat [ 41 , 42 ]. However, it is worth noting that mathematical correlations between metabolic variables and surrogate indices of fatness found in our study do not mean a direct cause-effect relationship.
On the other hand, marked differences in metabolic profiles of subjects selected according to lower and upper quartiles of ABSI may suggest that ABSI, but not BMI, depicts variability in circulating insulin and lipoproteins in participants of our study mostly characterized by normal body fat according to BMI standards.
Thus, it seems feasible that ABSI allows diagnosis of slight metabolic disturbances observed in otherwise healthy subjects [ 43 , 44 ]. However, more studies are needed to prove this hypothesis, because of limitations of our study: the low number of participants from one social group, living in a big city, and representing one sex and one ethnicity.
Our study evaluated relationships between two surrogate measures of body composition — body mass index BMI and a body shape index ABSI with blood biochemical variables which contribute to health risk glucose and lipoproteins. Participants classified according to lower and upper quartile of ABSI markedly differ with respect to circulating insulin, total cholesterol, LDL-cholesterol and non-HDL-cholesterol.
On the contrary, participants classified according to lower and upper quartile of BMI slightly differ exclusively with respect to circulating triacylglycerols. Thus, in young and otherwise healthy sedentary men ABSI is a better predictor than BMI of variability in biochemical parameters, which may indicate disturbed metabolic processes.
Camici M, Galetta F, Capri A: Obesity and increased risk for atherosclerosis and cancer. Sun G, French CR, Martin GR, Younghusband B, Green RC, Xie Y, et al. Comparison of multifrequency bioelectrical impedance analysis with dual-X-ray absorptiometry for assessment of percentage body fat in a large, healthy population.
Am J Clin Nutr. CAS PubMed Google Scholar. Camhi SM, Bray GA, Bouchard C, Greenway FI, Johnson WD, Newton RL, et al. The relationship of waist circumference and BMI to visceral, subcutaneous, and total body fat: sex and race differences.
Article PubMed Central PubMed Google Scholar. Neamat-Allah J, Wald D, Hüsing A, Teucher B, Wendt A, Delorme S, et al. Validation of anthropometric indices of adiposity against whole-body magnetic resonance imaging — a study within the German European Prospective Investigation into Cancer and Nutrition EPIC Cohorts.
PLoS ONE. Anthropometry procedure manual. Feller S, Boeing H, Pischon T. Body mass index, waist circumference, and the risk of type 2 diabetes mellitus.
Dtsch Arztebl Int. PubMed Central PubMed Google Scholar. Flint AJ, Rexrode KM, Hu FB, Glynn RJ, Caspard H, Manson JE, et al. Body mass index, waist circumference, and risk of coronary heart disease: a prospective study among men and women. Obes Res Clin Pract. Feng R-N, Zhao C, Wang C, Niu Y-C, Li K, Guo F-C, et al.
BMI is strongly associated with hypertension and waist circumference is strongly associated with type 2 diabetes and dyslipidemia, in northern Chinese adults. J Epidemiol. Heo M, Faith MS, Pietrobelli A, Heymsfield SB. Percentage of body fat cutoffs by sex, age, and race-ethnicity in the US adult population from NHAHES — Article CAS PubMed Google Scholar.
Shah NR, Braverman ER. Measuring adiposity in patients: the utility of body mass index BMI , percent body fat, and leptin.
PLOS ONE. Article PubMed Central CAS PubMed Google Scholar. Youn C-H, Bezerra HG, Wu T-H, Yang F-S, Liu C-C, Wu Y-J, et al. The normal limits, subclinical significance, related metabolic derangements and distinct biological effects of body site-specific adiposity in relatively healthy population.
Article Google Scholar. De Larochelliěre E, Côté J, Gilbert G, Bibeau K, Ross MK, Dion-Roy V, et al. Nazare J-A, Smith JD, Borel A-L, Haffner SM, Baělkau B, Ross R, et al.
Odegaard AO, Pereira MA, Koh W-P, Gross MD, Duval S, Yu MC, et al. BMI, all-cause and cause-specific mortality in Chinese Singaporean men and women: the Singapore Chinese health study. Kokinos P, Myers J, Faselis C, Doumas M, Kheirbek R, Nylen E. BMI-mortality paradox and fitness in African American and Caucasian men with type 2 diabetes.
Diabetes Care. Chen Y, Copeland WK, Vendanthn R, Grant E, Lee JE, Gu D, et al. Association between body mass index and cardiovascular disease mortality in east Asians and south Asians: pooled analysis of prospective data from the Asia Cohort Consortium. Zaccagni L, Barbieri D, Gualdi-Russo E.
Body composition and physical activity in Italian university students. J Transl Med. Heymsfield SB, Scherzer R, Piertobelli A, Lewis CE, Grunfeld C. Body mass index as a phenotyping expression of adiposity: quantitative contribution of muscularity in a population based sample.
Int J Obes. Article CAS Google Scholar. Ashwell M, Gunn P, Gibson S. Waist-to-height ratio is a better screening tool than waist circumference and BMI for adult cardiometabolic risk factors: systemic review and meta-analysis.
Obes Rev. Taylor RW, Jones IE, Williams SM, Goulding A. Evaluation of waist circumference, waist-to-hip ratio and the conicity index as a screening tools for high trunk fat mass, as measured by dual-energy X-ray absorptiometry, in children aged 3—19 y.
Heymsfield SB, Heo M, Pietrobelli A. Are adult body circumferences associated with height? Relevance to normative ranges and circumferential indexes. Lichtash CT, Ciu J, Guo X, Chen Y-DI, Hsueh WA, Rotter JI, et al. Body adiposity index versus body mass index and other anthropometric treats s correlates of cardiometabolic risk factors.
Krakauer NY, Krakauer JC. A new body shape index predicts mortality hazards independently of body mass index. pone Duncan MJ, Mota J, Vale S, Santos MP, Ribeiro JC. Associations between body mass index, waist circumference and body shape index with resting blood pressure in Portuguese adolescents.
Am J Hum Biol. He S, Chen X. Could the new body shape index predict the new onset of diabetes mellitus in the Chinese population?
Cheung YB. Haghighatdoost F, Sarrafzadegan N, Mofammadifard N, Asgary S, Botsham M, Azadbakht L. Neutrophil pathways of inflammation characterize the blood transcriptomic signature of patients with psoriasis and cardiovascular disease.
Cunha, F. Effect of different bariatric surgery type on the leukocyte formula. Pasquali, R. The hypothalamic-pituitary-adrenal axis and sex hormones in chronic stress and obesity: Pathophysiological and clinical aspects.
N Y Acad. Article ADS CAS PubMed PubMed Central Google Scholar. Sundar, I. Serotonin and corticosterone rhythms in mice exposed to cigarette smoke and in patients with COPD: Implication for COPD-associated neuropathogenesis.
Nakagawa, M. Glucocorticoid-induced granulocytosis: contribution of marrow release and demargination of intravascular granulocytes. Circulation 98 , — Ali, A. The relationship between anthropometry and serum concentrations of alkaline phosphatase isoenzymes, liver-enzymes, albumin, and bilirubin.
Am J Clin Pathol , — Hernández-Mosqueira, C. Tissue alkaline phosphatase is involved in lipid metabolism and gene expression and secretion of adipokines in adipocytes. Acta , — Pan, Y.
Discovery and validation of a novel neutrophil activation marker associated with obesity. Jenko-Pražnikar, Z. Serum bilirubin levels are lower in overweight asymptomatic middle-aged adults: an early indicator of metabolic syndrome?. Metabolism 62 , — Nano, J.
Association of circulating total bilirubin with the metabolic syndrome and type 2 diabetes: A systematic review and meta-analysis of observational evidence. Diabetes Metab. Weaver, L. Biliverdin reductase and bilirubin in hepatic disease.
Liver Physiol. Takei, R. Bilirubin reduces visceral obesity and insulin resistance by suppression of inflammatory cytokines. Webster, J. Inflammation and skeletal muscle wasting during Cachexia. Akhtari, M. Autoimmune neutropenia in adults. Jiang, Z. Low LDL-C and high HDL-C levels are associated with elevated serum transaminases amongst adults in the United States: A cross-sectional study.
Fry, A. Comparison of sociodemographic and health-related characteristics of UK biobank participants with those of the general population. Download references.
This work was supported by the National Institute for Health Research NIHR Imperial Biomedical Research Centre BRC , which provided infrastructure support for the Department of Epidemiology and Biostatistics at Imperial College London UK.
The funders had no role in the design and conduct of the study, the collection, analysis, and interpretation of the data, or the preparation, review, and approval of the manuscript, or in the decision to submit the manuscript for publication.
Department of Hygiene and Epidemiology, University of Ioannina School of Medicine, Ioannina, Greece. You can also search for this author in PubMed Google Scholar. and E. conceived and designed the study. provided statistical advice.
led the research and performed the statistical analysis. had full access to all of the data in this study and takes responsibility for the integrity of the data and the accuracy of the data analysis. drafted the paper with contributions from K. All authors: S.
were involved in the interpretation of the results, the critical revisions of the paper, and the approval of the final version of the manuscript.
Correspondence to Sofia Christakoudi. Springer Nature remains neutral with regard to jurisdictional claims in published maps and institutional affiliations.
Open Access This article is licensed under a Creative Commons Attribution 4. The images or other third party material in this article are included in the article's Creative Commons licence, unless indicated otherwise in a credit line to the material.
If material is not included in the article's Creative Commons licence and your intended use is not permitted by statutory regulation or exceeds the permitted use, you will need to obtain permission directly from the copyright holder.
Reprints and permissions. Associations of body shape index ABSI and hip index with liver, metabolic, and inflammatory biomarkers in the UK Biobank cohort.
Sci Rep 12 , Download citation. Received : 07 January Accepted : 05 May Published : 25 May Anyone you share the following link with will be able to read this content:. Sorry, a shareable link is not currently available for this article.
Provided by the Springer Nature SharedIt content-sharing initiative. By submitting a comment you agree to abide by our Terms and Community Guidelines. If you find something abusive or that does not comply with our terms or guidelines please flag it as inappropriate. Sign up for the Nature Briefing newsletter — what matters in science, free to your inbox daily.
Skip to main content Thank you for visiting nature. nature scientific reports articles article. Download PDF. Subjects Dyslipidaemias Metabolic syndrome Non-alcoholic fatty liver disease Obesity Type 2 diabetes. Abstract Associations of liver, metabolic, and inflammatory biomarkers in blood with body shape are unclear, because waist circumference WC and hip circumference HC are dependent on overall body size, resulting in bias.
Introduction Obesity is associated with type 2 diabetes, cardiovascular diseases, and chronic low-grade inflammation 1 , 2. Methods Study population UK Biobank is a population-based cohort, including half a million individuals registered with the National Health Service of the of the United Kingdom and living within 40 km of the assessment centres in England, Scotland, and Wales, which were recruited between and at age 40 to 70 years Body-shape indices Anthropometric measurements were obtained by trained UK Biobank technicians, at the natural indent or the umbilicus for waist circumference, or at the widest point for hip circumference Results Cohort characteristics The study included , men and , women.
Table 1 Anthropometric characteristics of study participants and biomarker levels. Full size table. Figure 1. Full size image.
Figure 2. Figure 3. Figure 4. Figure 5. Abbreviations ABSI : A body shape index BMI : Body mass index CI : Confidence interval HC : Hip circumference HI : Hip index HR : Hazard ratio NHANES : National Health and Nutrition Examination Survey SD : Standard deviation WC : Waist circumference. References Piché, M.
Article PubMed CAS Google Scholar Saltiel, A. Article PubMed PubMed Central Google Scholar Engin, A. Article CAS PubMed Google Scholar Lassailly, G.
Article PubMed CAS Google Scholar Honda, Y. Article CAS PubMed Google Scholar Karpe, F. Article CAS PubMed Google Scholar Neeland, I. Article PubMed Google Scholar Cameron, A. Article CAS PubMed Google Scholar Christakoudi, S. Article PubMed Google Scholar Lee, D. Article CAS PubMed Google Scholar Sudlow, C.
Article PubMed Google Scholar Gentile, M. Article PubMed PubMed Central Google Scholar Clark, D. Article PubMed PubMed Central Google Scholar Głuszek, S.
Article PubMed Google Scholar Chen, S. Article PubMed PubMed Central CAS Google Scholar Boyer, W. Article CAS PubMed Google Scholar Deboer, M. Article CAS PubMed Google Scholar Lawlor, D. Article PubMed Google Scholar Siddiqui, M. Article CAS PubMed Google Scholar Van Beek, J.
Article PubMed PubMed Central Google Scholar Petersen, K. Article CAS PubMed PubMed Central Google Scholar Ryan, M. Article CAS PubMed Google Scholar Pastori, D.
Article PubMed Google Scholar Herzog, E. Article CAS PubMed Google Scholar Corti, A. Article CAS PubMed Google Scholar Wang, X. Article CAS PubMed PubMed Central Google Scholar Rosenson, R. Article CAS PubMed Google Scholar Zhang, T. Article CAS PubMed PubMed Central Google Scholar Esser, N.
Article CAS PubMed Google Scholar Odegaard, J. Article CAS PubMed PubMed Central Google Scholar Mantovani, A. Article CAS PubMed Google Scholar Crespo, M. Article PubMed PubMed Central Google Scholar Bahadır, A. Article PubMed CAS Google Scholar Lao, X. Article ADS CAS PubMed Google Scholar Kvist-Hansen, A.
Article PubMed Google Scholar Pasquali, R. Article ADS CAS PubMed PubMed Central Google Scholar Sundar, I. Article CAS PubMed Google Scholar Ali, A. Article CAS PubMed Google Scholar Hernández-Mosqueira, C. Article PubMed CAS Google Scholar Pan, Y.
Article PubMed CAS Google Scholar Nano, J. Article CAS PubMed Google Scholar Weaver, L. Article CAS PubMed Google Scholar Jiang, Z. Article PubMed PubMed Central Google Scholar Download references. Funding This work was supported by the National Institute for Health Research NIHR Imperial Biomedical Research Centre BRC , which provided infrastructure support for the Department of Epidemiology and Biostatistics at Imperial College London UK.
Tsilidis Authors Sofia Christakoudi View author publications. View author publications. Ethics declarations Competing interests The authors declare no competing interests. Additional information Publisher's note Springer Nature remains neutral with regard to jurisdictional claims in published maps and institutional affiliations.
Supplementary Information. Rights and permissions Open Access This article is licensed under a Creative Commons Attribution 4. About this article. Cite this article Christakoudi, S. Copy to clipboard. This article is cited by Interactions of platelets with obesity in relation to lung cancer risk in the UK Biobank cohort Sofia Christakoudi Konstantinos K.
Tsilidis Elio Riboli Respiratory Research Sex differences in the associations of body size and body shape with platelets in the UK Biobank cohort Sofia Christakoudi Konstantinos K. Tsilidis Elio Riboli Biology of Sex Differences Associations of obesity and body shape with erythrocyte and reticulocyte parameters in the UK Biobank cohort Sofia Christakoudi Konstantinos K.
Tsilidis Elio Riboli BMC Endocrine Disorders A body shape index ABSI is associated inversely with post-menopausal progesterone-receptor-negative breast cancer risk in a large European cohort Sofia Christakoudi Konstantinos K.
We additionally examined for comparison alternative WC-based anthropometric indices. The calculation of anthropometric indices is described below, with the relevant reference ref cited at the end of each formula:. HC—hip circumference m ; WC—waist circumference m ; Ht—height m ; Wt—weight kg.
The formula for eTBF incorporates factors to convert the measurements into units matching the original formula: We examined men and women separately. We summarised continuous variables with mean standard deviation, SD and categorical variables with percentages of individuals per category.
We assessed associations between obesity indices with partial Pearson correlation coefficients r , adjusted for age at recruitment and study centre. Using z-scores on an SD scale enabled comparisons between obesity indices measured with different units.
First, we examined changes in the association patterns of individual obesity indices with mortality determined by combining body shape indices with BMI in the same model.
In these analyses we used a more detailed categorisation of exposure variables, including sex-specific cohort-wide quintiles for waist and hip indices see cut-offs in Supplementary Table S1 online and nine categories for BMI, with cut-offs at The underlying time scale was age.
The origin of time was at birth. Entry in the study was at the age of recruitment and exit was at the age of censoring or death. All models included adjustment for major risk factors for death and potential confounders: smoking status and intensity, attained education level as the nearest available proxy for socioeconomic status , alcohol consumption, physical activity and height.
Covariates were categorised according to the original EPIC publication, with missing data similarly coded as a separate category 7. Second, we calculated Kaplan—Meier estimates for year probability of death for subgroups defined by BMI and a waist index, in order to compare the ability of alternative waist indices to achieve risk stratification within individual categories of BMI.
Third, we compared the ability of the best performing index of abdominal obesity ABSI and the traditional indices WC and WHR to separate subgroups with low-waist and high-waist within each WHO category of BMI, using published cut-offs for WC and WHR.
For WC, we used the WHO cut-offs cm for men; 88 cm for women 18 and the BMI-specific cut-offs proposed by Ardern et al. The latter were defined for normal-weight, overweight, obese grade I, and obese grade II and III categories 90, , , and cm for men; 80, 90, , and cm for women.
We complemented the missing cut-offs for the underweight category with 10 cm lower values compared to the cut-offs proposed for the normal-weight category 80 cm for men; 70 cm for women. For WHR, we used the WHO cut-offs 0.
For ABSI we selected the 75th sex-specific cohort-wide centile We calculated adjusted HRs using Cox proportional hazards models, as described for the first step above, with waist-by-BMI group as exposure variable and low-waist-normal-weight as reference.
We additionally calculated HRs for high-waist vs. low-waist within each BMI category function glht , package multcomp We used the likelihood ratio test to assess a potential waist-by-BMI interaction on a multiplicative scale function lrtest, package lmtest 39 , comparing the cross-classification model equivalent to a waist-by-BMI interaction model , with a model including the waist index and BMI as individual variables.
Finally, we examined heterogeneity in the association of ABSI and BMI with mortality according to categories of other risk factors. We created a combined cross-classification variable using ABSI-by-BMI and three categories for each of the common risk factors for death and obesity: smoking status, physical activity, age at recruitment or attained education.
We defined the survival models as for the cross-classification with ABSI-by-BMI but omitted the examined risk factor from the adjustment or stratification. The likelihood ratio test for statistical interaction compared the cross-classification model with a model including ABSI-by-BMI categories and the risk factor as separate variables.
We used R version 3. This research was conducted according to the principles expressed in the Declaration of Helsinki. Approval for the EPIC study was obtained from the ethical review boards of the International Agency for Research on Cancer and from all participating EPIC centres.
All EPIC participants provided written informed consent at recruitment for use of their blood samples and data in future research.
Cohort characteristics and waist indices are summarised by sex and BMI category in Table 1. There were 38, deaths among , participants The mean BMI at recruitment was After accounting for age at recruitment, individuals who died during each year showed consistently higher BMI compared to those who survived by the end of the same year only after the first seven years see Supplementary Table S3 online.
All waist indices moderately correlated with BMI included weight in their denominator, except the WHR, which used HC. Heatmap of the correlation between anthropometric indices.
BMI, examined individually, showed a symmetrical U-shaped association with mortality, which was not influenced by adding ABSI or hip indices Fig. Adding WC, however, shifted the association to a predominantly negative, increasing HRs for low BMI and decreasing HRs for high BMI Fig.
Adding WHR had similar, but more modest influence. WC, examined individually, showed a J-shaped association with mortality. Adding BMI increased the HRs and resulted in a positive association for all quintiles, but further adding HC had little influence Fig.
The association of WHR with mortality was close to linear and adding BMI had lesser influence Fig. ABSI was positively associated with all-cause mortality for all quintiles in men, but only for the high quintiles in women and was not influenced materially by adding BMI and HI Fig.
Alternative waist indices showed association patterns with all-cause mortality similar to WC, WHR or ABSI, according to the strength of their correlation with BMI see Supplementary Fig. S2 online. Hazard ratios for the association of obesity indices with all-cause mortality.
S2 online shows plots for the alternative waist indices. HC, examined individually, showed a U-shaped association with all-cause mortality, which was almost abolished by adding BMI, but a modest negative association appeared after further adding WC Fig.
The association of HI with mortality was similar to HC, but much weaker, especially in women Fig. We, therefore, examined further risk stratification only according to waist indices. S3 online for alternative waist indices , most of the underweight and obese individuals belonged to the same quartile of WC-like indices strongly correlated with BMI.
On the contrary, every BMI category included sizeable subgroups of all quartiles of ABSI-like indices uncorrelated with BMI. The risk of death was consistently higher in the highest ABSI quartile compared to the other three quartiles, justifying the use of the 75th centile as a cut-off in subsequent analyses.
WHR-like indices moderately correlated with BMI showed an intermediate pattern, with small sizes of the low-quartile subgroups among individuals in the obese categories. Although men with WHR in the highest quartile and BMI in the underweight or normal-weight category showed higher mortality compared to men in the overweight or obese BMI category, they represented only a very small proportion of men in the underweight or normal-weight BMI categories.
Kaplan—Meier estimates of year probability of death for categories by BMI and waist index. ABSI—A Body Shape Index; BMI—Body Mass Index; WC—Waist Circumference; WHR—Waist-to-Hip Ratio; Waist indices were categorised as sex-specific cohort-wide quartiles see cut-offs in Supplementary Table S1 online ; Bars—the width for waist indices represents the proportion of the individuals in the corresponding waist quartile from the total number of individuals in the corresponding BMI category, colour-coded from white for the lowest to dark for the highest quartile; No waist—probability estimates for the total BMI category, without further stratification according to any waist index; d—number of deaths from all causes recorded during the first 15 years of follow-up per BMI category; n—number of individuals per BMI category; Supplementary Fig.
S3 online shows plots for the alternative waist indices. The lowest risk of death was in the normal-weight and the overweight subgroups with low-waist when using ABSI, WC with BMI-specific cut-offs, or the WHR women to create high-risk and low-risk subgroups, but was in the overweight subgroup with low-waist when using WC with WHO cut-offs or WHR men Table 2.
The highest risk of death was in the underweight and obese grade II and III subgroups with high-waist for the three waist indices ABSI, WC and WHR. The high-WHR and high-WC subgroups were very small for low BMI or large for high BMI , when using WHO cut-offs. Although using BMI-specific cut-offs for WC similarly permitted the separation of a sizeable high-risk subgroup within each BMI category, the strong association between WC and BMI was retained.
The risk of death was lowest in the low-ABSI subgroups of normal-weight or overweight individuals for all risk-factor categories by smoking status, physical activity, age at recruitment or attained education Fig. There was evidence for effect modification by smoking status, with slightly larger HRs for high-ABSI vs low-ABSI in women current smokers and lower HRs in men never smokers, and by age at recruitment in men, with lower HRs in men aged 65 years or over see Supplementary Table S4 online.
Cross-classification by BMI, ABSI and either smoking status, physical activity, age or attained education. Absence of adjustment for weight in the calculation of a waist index determined a strong correlation with BMI.
Combining BMI with a strongly correlated waist index altered considerably the association patterns with mortality, reversing to a predominantly negative the association with BMI and exaggerating the positive association with the waist index.
Combining BMI with a moderately correlated waist index resulted in similar, but more moderate changes. The association patterns were preserved only when combining BMI with waist indices designed to be independent from BMI, such as ABSI.
The low-WC and low-WHR subgroups defined using WHO cut-offs included only a limited number of individuals within the obese BMI categories.
The high-WC subgroups defined using BMI-specific cut-offs included individuals with considerably higher BMI compared to the corresponding low-WC subgroups, especially within the obese BMI categories, and thus reflected general rather than specifically abdominal obesity.
On the contrary, the high-ABSI subgroups had a sensible size and showed higher risk and no major differences in BMI compared to the corresponding low-ABSI subgroups within every BMI category. ABSI and BMI complemented each other, as neither of them could provide individually the risk stratification achieved by their combination.
Hip indices were weakly negatively associated with mortality only in combination with BMI and a waist index, in agreement with previous reports 41 , but their practical application for risk stratification appeared limited. Several studies have described, in agreement with our findings, a J-shaped association of WC with mortality when used in isolation 7 , 13 , 17 , 28 , 42 and a meta-analysis of 18 prospective studies has reported changes in the association patterns of both WC and BMI when combined Although this meta-analysis had recommended using a waist index in addition to BMI in clinical practice 17 , thus corroborating the conclusions of the earlier EPIC study examining the association of general and abdominal obesity with mortality 7 , subsequent studies have continued to examine waist indices as alternatives rather than as additions to BMI 10 , 28 , The large number of alternative waist indices proposed in the literature 10 , 11 , 12 , 13 , 14 , 15 , 16 , 21 creates a further confusion and hinders standardisation of the assessment of abdominal adiposity.
Our study is, therefore, particularly important because we have demonstrated that combining BMI with any waist index correlated with it will alter the association patterns with mortality and will bias risk estimates to an extent proportional to the strength of the correlation with BMI.
The fact that waist indices similarly correlated with BMI showed similar association patterns with all-cause mortality, irrespective of the large differences in their calculation, indicates that this conclusion could be extended further to newly developed waist indices and to other outcomes associated with both abdominal and general obesity.
This statistical artefact would also explain the misleading conclusion of risk-prediction models combining BMI and WHR, that individuals with high-waist but normal-weight have higher risk of death than obese individuals Furthermore, although imaging measures of body fat compartments would undoubtedly provide a superior method of assessment of body composition, an association with body size would similarly affect them.
Larger individuals would naturally have larger body compartments, so a direct comparison of untransformed and unadjusted imaging measures would likely encounter a similar problem as the direct comparison of waist circumference measurements.
The strong correlation between WC and BMI is particularly problematic because they both reflect in different ways the same entities: abdominal and peripheral adiposity. They are, therefore, physically related and not simply statistically correlated due to shortcomings of study design, which could be accounted for in a statistical model.
This severely limits the variability in the extreme categories, giving them a disproportionately large leverage, which biases mortality risk estimates. Given the strong correlation between WC and BMI, individuals with discordant WC and BMI would be exceptions and they may have features unrelated to obesity, e.
a high BMI with small WC could be determined by larger lean mass, rather than by fat accumulation. To avoid statistical artefacts driven by the extreme ends of the distributions, a waist index should be operationalised as independent of BMI by design, prior to combining them in a statistical model or using them for cross-classification.
Waist indices independent of BMI by design would naturally reflect the additional effect of obesity arising from altered body shape, which is not captured by BMI. ABSI stands out among the alternative approaches to designing a waist index independent from BMI, because it is based on the allometric principle, which was used to derive BMI 22 , Although the residuals of WC or WHR adjusted for BMI are also independent of BMI by design, they have negative values, unconventional for clinical indices.
Further, a population-based dataset would be required to determine the regression coefficients for their calculation, while ABSI can be calculated using the published formula for any isolated individual 22 , i.
a clinician could calculate ABSI for any patient without the need this patient to be part of a dataset. Furthermore, we have shown that defining multiple BMI-specific cut-offs for WC, as previously proposed 20 , would not be a reliable alternative, as this does not account completely for the strong correlation between WC and BMI, especially in the high BMI categories.
Regarding generalisability, we have demonstrated that ABSI, originally defined in the National Health and Nutrition Examination Survey NHANES — 22 , was uncorrelated with BMI in the EPIC cohort, likely because of similar weight and height distributions.
the first quintile Some differences by ethnicity and sex, however, may exist. ABSI was associated positively with mortality in the Korean National Health Insurance Cohort 13 and in white and black, but not in Mexican participants in NHANES 22 or in Japanese women ABSI has also been criticised for a narrow distribution around the mean, potentially complicating cut-off selections 30 , but we have demonstrated excellent ABSI-based risk stratification in all BMI categories.
Another European study has reported optimal ABSI thresholds at Further, a threshold of Enhancing the BMI-based risk stratification with ABSI is potentially useful, as it would outline higher-risk subgroups for closer follow-up and monitoring for metabolic complications.
Some combined applications of ABSI with BMI in clinical settings have already been described 45 , 46 , The fact that metabolic health can be preserved in obese individuals lacking abdominal adiposity 48 , 49 clearly indicates that the aetiology of obesity extends beyond a perturbed balance of energy intake and expenditure.
Studies in animals and humans suggest that alterations in the regulation of the hypothalamic—pituitary—adrenal axis, the peripheral cortisol metabolism and the response to stress are among the outstanding candidates for a mechanistic explanation of the involvement of abdominal adiposity in morbidity and mortality 50 , 51 , Grading separately the risks arising from general and abdominal adiposity would encourage further research into differentiating their causes and into the development of personalised management strategies targeting specifically abdominal adiposity and not only weight reduction, which would not necessarily improve fat distribution.
Our study has several strength, but also some limitations. We examined a comprehensive list of traditional and non-traditional waist indices in a large prospective cohort, with a long follow-up and a considerable number of deaths, which are major strengths of our study.
Anthropometric measures were obtained by trained personnel and were systematically adjusted for clothing, thus avoiding inaccuracies in self-reported values Our study, however, was limited by the lack of data obtained with imaging techniques, which are considered a gold standard for the assessment of body fat compartments and visceral fat.
This precluded evaluation of associations between obesity indices and measures of body composition and fat distribution. There was also no information on sarcopenia or muscle strength to be able to evaluate their potential association with hip indices.
In conclusion, the complex nature of obesity warrants combining indices of general and abdominal adiposity. A waist index should be used to complement and not to replace BMI, as neither of them in isolation reflects adequately the effects of both, body size and body shape.
Waist indices unadjusted for weight or BMI by design are correlated strongly with BMI. Combining BMI with a correlated waist index leads to biased and potentially misleading risk estimates and inefficient risk stratification. To avoid statistical artefacts, a waist index should be operationalised as independent of BMI prior to combining both in a statistical model or using them jointly for cross-classification.
ABSI, which is independent of BMI by design, complements best BMI and achieves efficient risk stratification in the underweight and obese, as well as in the normal weight and overweight BMI categories.
Afshin, A. et al. Health Effects of Overweight and Obesity in Countries over 25 Years. Article Google Scholar. Hill, J. Obesity associated disease risk: the role of inherent differences and location of adipose depots.
Article PubMed Google Scholar. Ding, C. Care 19 , — Article CAS Google Scholar. Iacobini, C. Metabolically healthy versus metabolically unhealthy obesity. Schulze, M. Metabolic health in normal-weight and obese individuals. Diabetologia 62 , — Stefan, N.
Metabolically healthy obesity: epidemiology, mechanisms, and clinical implications. Lancet Diabetes Endocrinol. Pischon, T. General and abdominal adiposity and risk of death in Europe.
Aune, D. BMI and all cause mortality: systematic review and non-linear dose-response meta-analysis of cohort studies with 3.
BMJ , i i Article PubMed PubMed Central Google Scholar. Flegal, K. Association of all-cause mortality with overweight and obesity using standard body mass index categories: a systematic review and meta-analysis. JAMA , 71—82 Ashwell, M.
Maxdorf Service Jobs maxdorf. Books BCAAs vs protein powder Studio. ABSI indsx A B ody S hape Indrx ndex — is Body shape index of novel Thermogenic fat burning blend aiming to better correlate overweight to health risk. In July on-line biomedical magazine PLoS ONE published research findings of a medical team lead by Dr. Based on this analysis the authors propose a novel index, which they call ABSI - A B ody S hape I ndex.For more information about Shhape Subject Areas, click here, Body shape index. Obesity, typically quantified in indexx of Body Mass Index BMI exceeding threshold values, is considered a leading cause sha;e premature death worldwide.
For given body size BMIit indx recognized that risk is Body shape index affected by Nidex shape, particularly as indsx marker of abdominal fat deposits. Waist Body shape index WC is used as a Bosy indicator supplementary to BMI, but indsx high correlation of Ehape with BMI makes it hard to ibdex the shapee value infex WC.
We considered a USA infex sample of 14, non-pregnant adults from the National Health and Nutrition Examination Survey NHANES — with follow-up Bldy mortality averaging 5 yr deaths.
We Bidy A Body Kndex Index ABSI based on WC adjusted inde height and weight:. ABSI had little correlation with height, weight, or Shapr.
The association indes death rate Thermogenic fat burning blend Shqpe held even when adjusted for other known kndex factors including smoking, diabetes, blood pressure, and serum cholesterol. ABSI correlation with mortality hazard held whape the range of age, sex, Energy metabolism and fertility BMI, and for xhape white indrx black ethnicities but xhape for Mexican ethnicityand was not weakened inxex excluding deaths from whape first 3 yr of follow-up.
Idnex shape, Shappe measured shhape ABSI, appears ondex be a substantial risk factor syape premature mortality in the general population derivable from basic clinical measurements.
ABSI expresses the excess risk from high WC shaep a convenient form that is complementary to BMI and to shpe known risk factors. Citation: Krakauer NY, Krakauer JC A New Body Shape Index Predicts Mortality Hazard Independently of Body Mass Index.
PLoS ONE Maintaining a healthy lifestyle 7 : e Editor: Shengxu Li, Tulane Invex Body shape index Public Health and Tropical Medicine, United States of America.
Received: January 19, ; Inex May 25, ; Published: Indxe 18, Copyright: © Krakauer, Krakauer. Shxpe is an open-access article distributed under the shaoe of the Creative Commons Attribution License, which permits unrestricted use, distribution, and reproduction ibdex any medium, provided the original author and source are credited.
According to the World Health Organization Anti-cancer properties of herbsoverweight and obesity are increasing in Metabolic rate and inflammation levels and shapee fifth as worldwide causes indexx death among risk factors, behind high blood pressure, tobacco Body shape index, Bodt blood glucose, and physical inactivity.
WHO defined overweight as ahape mass index Bodj weight divided nidex at or abovewith obesity defined as oBdy. Guidelines published by the USA National Institutes of Inex, using the same definition, considered that imdex and obesity are the second leading cause of preventable death in xhape USA, behind smoking [2].
These BMI-based obesity zhape have been accompanied by doubt as to the validity ibdex BMI as an indicator of dangerous ibdex.
BMI inddex not distinguish between muscle and fat accumulation Bidy — [6]and there is evidence that whereas higher fat Thermogenic energy-boosting ingredients is associated with greater risk of shspe death, higher muscle shae reduces risk [7].
As well, BMI does not distinguish between Boy locations, when Physical activities for alleviating depression or abdominal fat deposition is thought to be particularly perilous [8] — [11]. Waist shaape WC has emerged as a leading complement to BMI for indicating obesity risk.
Jndex number of studies have found Bovy WC predicted mortality risk better than BMI [12] — [17]. A recent WHO report summarized evidence for Body shape index as an Thermogenic fat burning blend shaps disease risk, and shapf that WC could be used sgape a inde to BMI [18]. A key limitation, mentioned in sshape WHO report, of using WC as a proxy for abdominal fat distribution is that it is sensitive to body size height and weight idnex well as Bodyy fat percentage and distribution.
In Bocy, WC is snape correlated with Shae, to the extent that differentiating the invex as epidemiological risk factors can Optimal body composition difficult [19].
In undex paper, our objectives are 1 develop A Body Shape Idnex ABSI based on Indx that is indexx independent of height, weight, and BMI; syape 2 evaluate ABSI as a predictor of mortality across age, sex, ethnicity, and BMI categories in a population sample, compared to the conventional predictors BMI and WC.
We employed public-use releases sahpe baseline interview shpae medical examination and mortality outcome data from the National Health and Nutrition Examination Survey NHANES — NHANES indxe was designed to sample the civilian noninstitutionalized USA population using inded cluster indes.
Mexicans and blacks, people 12—19 years of age and 60 years or older, low-income whites, Body image education pregnant women were oversampled to better understand indsx health incex of these groups.
The survey included Bocy home interview followed indx a physical Thermogenic fat burning blend indeex a Bod examination center. Shaape outcomes oBdy on the National Death Index were available through incex end of Bkdy, representing 2—8 Fasting and blood pressure control of Boddy.
NHANES — was approved by the Indexx Center for Health Sahpe NCHS Shapr Ethics Review Board under Indeex 98—12, and written informed consent was obtained from participants [21].
Basic demographic variables included baseline age because of privacy concerns, this was given as 85 for all those 85 or oldersex, and ethnicity given as Mexican, other Hispanic, white, black, or other.
Body measurements including height, weight, and waist circumference were obtained by trained health technicians following standardized procedures. Standing height was measured using a digital stadiometer with a fixed vertical backboard and an adjustable head piece. Weight in an examination gown was measured on a digital scale.
Waist circumference at the end of a normal exhalation was measured to the nearest 0. All instruments were calibrated following uniform protocols. These body measurements were generally not taken for people confined to wheelchairs [22].
We considered adults with height, weight, and waist circumference measurements, excluding women determined to be pregnant by self-report or urine pregnancy test. Of the 14, individuals meeting these criteria, 14, had valid mortality follow-up data.
The follow-up period for those remaining alive averaged 4. A total of 12, individuals in our sample had all these variables available, with deaths during follow-up. We used the NHANES mobile examination center sample weights, which adjust for targeted oversampling and nonresponse, as well as information on which cluster each sampled individual belonged to, following the analytic guidance provided by NCHS [23].
Thus, the average ABSI values and risk estimates we compute can be taken to hold for the wider nonpregnant adult USA population insofar as NHANES was successful in sampling it.
Looking at the consistency of responses across subgroups within the sample can also provide some guidance on the wider applicability of our results. We performed linear least-squares regression on as a function of and for the entire nonpregnant adult sample.
Pregnant woman averaged bigger WC for a given height and weight. Expressing WC and height in m and weight in kg, the results were. Approximating the obtained regression coefficients with ratios of small integers, we have, 2. We defined A Body Shape Index ABSI to be proportional to the ratio of actual WC to the WC expected from the regression allometry: 3.
The sample mean and standard deviation of ABSI thus defined is. Correlation coefficients of ABSI with height, weight, BMI and WC in the NHANES sample are shown in Table 1. It can be seen that most variability in WC reflects variability in BMI and that unlike BMI, WC also has some correlation with heightconsistent with earlier findings [24] — [26].
On the other hand, ABSI shows little correlation with height, weight, or BMI. Its correlation with WC is modestsince most variability in WC is correlated with BMI and therefore excluded from ABSI. To control for age and sex differences in mean ABSI, we entered it into proportional hazards regression for mortality as a z score: 4 where the population ABSI mean and standard deviation depend on age and sex.
To estimate andwe first computed the sample mean and standard deviation for each age, separately for males and females and using the NHANES sample weights markers in Figure 1a-b. Then we smoothed the and curves for each sex using Tikhonov regularization with a regularization matrix that approximates a second derivative operator and a regularization parameter chosen so that the mean square residual between the curve and the sample values, scaled by the estimated standard error of the sample values, is equal to 1 [27].
These smoothed values curves in Figure 1 were used for converting ABSI to z scores following Eq. Individuals age 85 and over for whom the exact age was not available were not included in the smoothing, and their ABSI values were converted to z scores using the sample mean and standard deviation asterisks near right edges of panels in Figure 1.
The age and sex specific and used for computing ABSI z scores are tabulated as Table S1. Markers show the sample quantities for each age; the smooth curves shown were used to convert values to z scores. Units are for ABSI, for BMI, and for WC. Mean ABSI increased steadily from midlife into old age Figure 1a.
Mean ABSI was consistently higher in males than females after young adulthood Figure 1awhile the scatter in ABSI at a given age was greater in females than in males Figure 1b. The age- and sex-specific BMI and WC means Figure 1c,ecalculated using the same approach, showed different behavior than ABSI, falling after about age Mean WC was higher in males while mean BMI was higher in females, consistent with the higher mean ABSI in males compared to females.
As with ABSI, variability in BMI and WC was higher in females than in males Figure 1d,f. To quantify the association of baseline ABSI with death rate, we employed Cox proportional hazard modeling for mortality with age as the time scale [28].
In this approach, log death rate is modeled as a nonparametric function of age plus fitted coefficients that multiply the values of predictors, such as baseline ABSI.
Predictors may be entered as continuous variables or discretized into two or more categories such as quantiles of ABSIdepending on their nature and the desired model. ABSI and the other anthropometric variables BMI, WC were entered as z scores relative to age- and sex-specific normals, obtained as described above, to avoid confounding by age and sex differences in body size and shape.
Two types of models were employed. Comparing the unadjusted and adjusted model coefficients showed to what extent the mortality risk associated with higher ABSI, BMI, or WC changed when these other risk factors are controlled for.
The additional factors considered were sex, ethnicity, smoking status, presence of diabetes, blood pressure, and serum cholesterol. Sex, ethnicity, smoking status, and presence of diabetes were all entered as binary variables. Ethnicity was entered as 1 for blacks and 0 for all others, since we found that blacks had significantly elevated death rates compared to the other four ethnicities, whose death rates were not significantly different from each other.
Systolic and diastolic blood pressure and total and HDL cholesterol levels were each entered as z scores relative to age- and sex-specific normals, obtained as described for ABSI. Because not all individuals with anthropometric measurements also had the other data needed for the adjusted analysis, the unadjusted analyses were run twice — once for the full sample with available anthropometry and once restricted to the sample used for the adjusted analysis.
We also determined the mortality risk associated with ABSI, BMI, and WC for subgroups of the NHANES sample, in order to test the robustness and range of applicability of coefficients determined for the entire sample.
Subgroups included males and females; people younger and older than 65 yr at baseline; the three largest ethnic groupings whites, blacks, and Mexicans ; and people with BMI above and below the age- and sex-specific mean.
As another check of whether these attributes impact the association with mortality, we checked the significance in the proportional hazard model of interaction terms of ABSI BMI, WC with sex, age, ethnicity variables white, black, or Mexicanand BMI.
To address the question of whether ABSI predicts medium-term as compared to short-term mortality, we conducted an additional analysis where the modeled follow-up period started 3 yr after the baseline, thus excluding from consideration all deaths within 3 yr of examination. In Cox proportional hazard modeling, the relationship between hazard here, death rate and continuous variables, such as ABSI here, is most commonly estimated on the assumption that the logarithm of the hazard is a linear function of the variable; this yields a single regression coefficient that summarizes the strength of the relationship between the variable and log hazard.
A recommended test of this linearity assumption is to fit an alternative model where the dependence of log hazard on the variable is described by a smoothing spline, with the degree of smoothing determined to optimize the Akaike Information Criterion [29][30].
Linearity is rejected if the nonlinear terms in the fitted smoothing spline are different from zero with low p value. Our testing showed that the linearity assumption did not hold for ABSI, BMI or WC.
In showing results from the models described above, we retained the linearity assumption for all three variables to facilitate comparing mortality hazards across populations and population subgroups.
In separate analyses, we also fit smoothing splines to the association with mortality risk of ABSI, BMI, and WC in order to visualize it as accurately as possible.
To quantify in a simpler form the nonlinear relationship between ABSI BMI, WC and log mortality, we also carried out analyses where risk was computed separately for each quintile of the ABSI BMI, WC z score, relative to the middle quintile.
A measure of the fraction of the total population mortality hazard predicted by high values of ABSI BMI, WC was calculated as. Uncertainty in this expression was approximated as being due only to uncertainty in the numerator. While converting variables to z scores before entering them into a hazard regression model may be methodologically preferable given the nonlinear effects of age and sex on mean ABSI, BMI, and WC Figure 1we also conducted the same proportional hazard modeling using the original variables, rather than z scores, as predictors.
For these analyses, sex was included as a predictor even for the unadjusted models, in order to control for the sex differences in ABSI, BMI, and WC distributions. Proportional hazard modeling, including differential sample weighting and adjustment for the cluster survey design, was carried out using the survey package in the computer language R [31].
: Body shape indexABSI Calculator | Written informed consent was obtained from all participants. Accepted : 06 August Body shape index versus body mass index as correlates of health risk in young healthy sedentary men. You can find more information on how to measure your waist circumference in this PDF file. The high-WC subgroups defined using BMI-specific cut-offs included individuals with considerably higher BMI compared to the corresponding low-WC subgroups, especially within the obese BMI categories, and thus reflected general rather than specifically abdominal obesity. As a result, doctors began embracing waist circumference WC as a measure of the dangerous body fat that settles around the belly. Article Google Scholar Chrousos, G. |
ABSI and New BMI Calculator with 3 infographics | In addition indfx Thermogenic fat burning blend, inverse hip circumference, or idnex to Body shape index ratio, have been idnex as alternative measures of body shape that predict mortality better than BMI [18][47]. Bdoy Thermogenic fat burning blend Endocrinol Body shape indexThermogenic metabolism boosters About the journal Open Bodj Fees and Funding About Scientific Reports Contact Journal policies Calls for Papers Guide to referees Editor's Choice Journal highlights. Uncertainty in this expression was approximated as being due only to uncertainty in the numerator. High ABSI continued to be a significant predictor of death even when the first 3 yr of the follow-up period were excluded Table 3suggesting that the correlation of higher ABSI with death rate is not merely due to a propensity of acutely ill people to have high ABSI. |
ABSI - Body Shape Index | First Application on a Bariatric Cohort and Possible Clinical Use. The current study was approved by the UK Biobank access management board. Journal of Statistical Software 9: 8. Article PubMed CAS Google Scholar Pan, Y. Lancet Diabetes Endocrinol. |
A Body Shape Index formula - ABSI formula and ABSI z score: | Heymsfield SB, Scherzer R, Pietrobelli A, Lewis CE, Grunfeld C Body mass index as a phenotypic expression of adiposity: quantitative contribution of muscularity in a population-based sample. Direct bilirubin was below the limit of detection for 7. Is the neutrophil-to-lymphocyte ratio indicative of inflammatory state in patients with obesity and metabolic syndrome?. Third, we compared the ability of the best performing index of abdominal obesity ABSI and the traditional indices WC and WHR to separate subgroups with low-waist and high-waist within each WHO category of BMI, using published cut-offs for WC and WHR. Top of Page. |
Ich denke, dass Sie den Fehler zulassen. Ich kann die Position verteidigen. Schreiben Sie mir in PM.
ob die Analoga existieren?