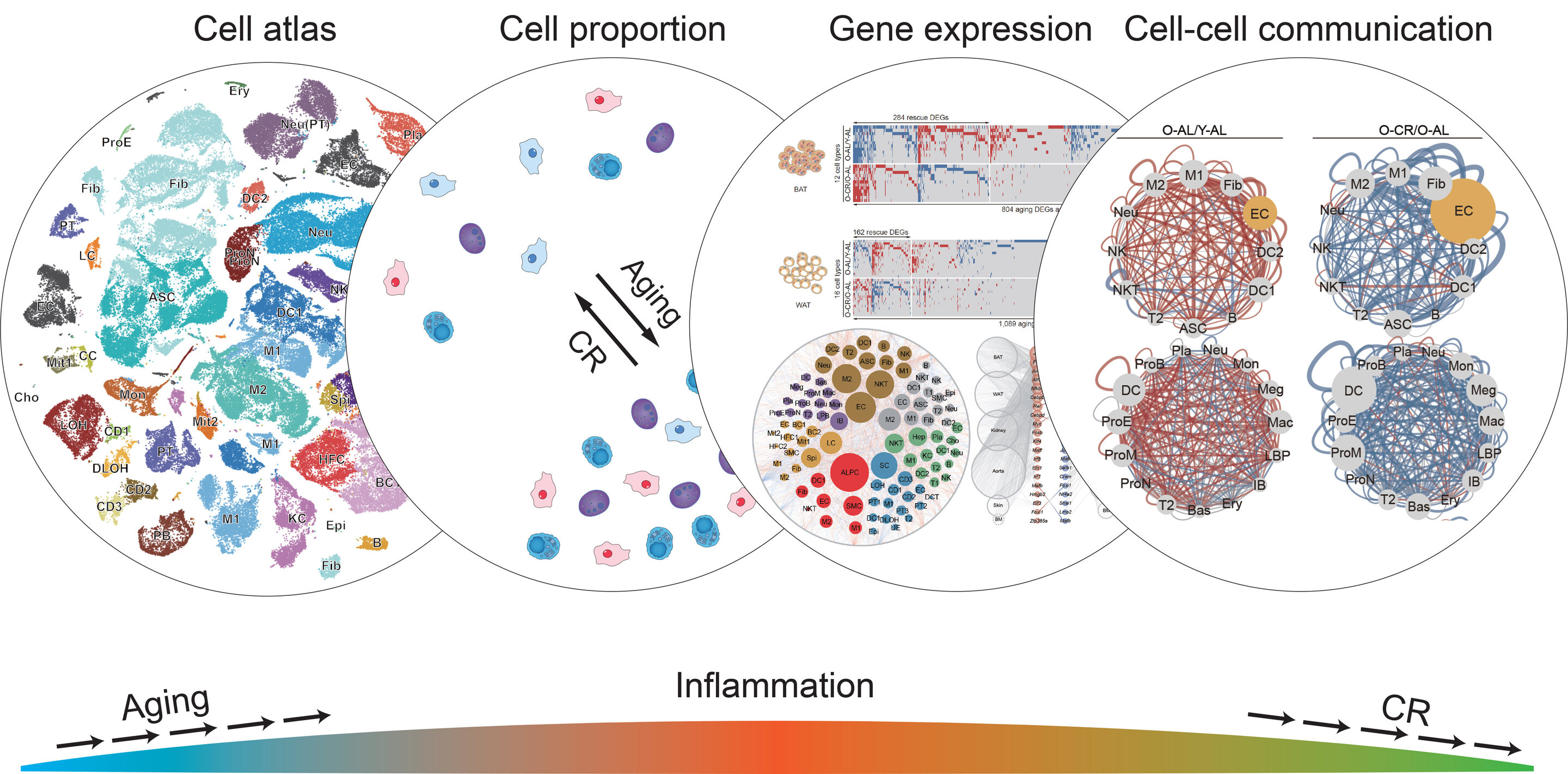
Caloric restriction and cellular health -
Aging 1 , 47—59 Davis, S. methylumi: handle Illumina methylation data. R package version 2. Huber, W. Orchestrating high-throughput genomic analysis with Bioconductor. Methods 12 , — Lehne, B.
A coherent approach for analysis of the Illumina HumanMethylation BeadChip improves data quality and performance in epigenome-wide association studies.
Aryee, M. Minfi: a flexible and comprehensive Bioconductor package for the analysis of Infinium DNA methylation microarrays. Bioinformatics 30 , — Horvath, S. DNA methylation-based biomarkers and the epigenetic clock theory of ageing. DNA methylation age of human tissues and cell types.
Hannum, G. Genome-wide methylation profiles reveal quantitative views of human aging rates. Cell 49 , — Chen, B. DNA methylation-based measures of biological age: meta-analysis predicting time to death. Aging 8 , — Assessment of epigenetic clocks as biomarkers of aging in basic and population research.
A 75 , — Quantification of biological aging in young adults. Natl Acad. USA , E—E Elliott, M. Disparities in the pace of biological aging among midlife adults of the same chronological age have implications for future frailty risk and policy.
Patterns of reliability: assessing the reproducibility and integrity of DNA methylation measurement. Patterns 1 , Stata Multilevel Mixed-Effects Reference Manual StataCorp, Sussman, J.
An IV for the RCT: using instrumental variables to adjust for treatment contamination in randomised controlled trials. Baum, C. Instrumental variables and GMM: estimation and testing.
Stata J. Bang, H. On estimating treatment effects under non-compliance in randomized clinical trials: are intent-to-treat or instrumental variables analyses perfect solutions?
Shapiro, S. An analysis of variance test for normality complete samples. Biometrika 52 , — Brown, M. Robust tests for the equality of variances. Markowski, C. Conditions for the effectiveness of a preliminary test of variance. Google Scholar. Download references. This research was supported by grant no.
R01AG to D. received additional support from the American Brain Foundation to R. and V. received additional support from grant no. P30AG to C. R01AG to V. and C. R33AG to K. received additional support from the CIHR grant no. RN to M. and S. received support from grant no. R01 AG to S. and W. R03AG to I.
U01AG to B. We thank the CALERIE Research Network no. R33AG for their assistance in this project and the Dunedin Study no. R01AG for facilitating early access to the DunedinPACE DNA methylation algorithm. The funders had no role in study design, data collection and analysis, decision to publish or preparation of the paper.
completed work on this project while affiliated with the Butler Columbia Aging Center. She is now in the Department of Neurology at the Columbia University Irving Medical Center. Butler Columbia Aging Center, Columbia University Mailman School of Public Health, New York, NY, USA.
Waziry, C. Ryan, M. Kothari, G. Department of Genetics, University of North Carolina at Chapel Hill School of Medicine, Chapel Hill, NC, USA. Duke Molecular Physiology Institute and Department of Medicine, Duke University School of Medicine, Durham, NC, USA. Huffman, V.
Department of Medical Genetics, Edwin S. Leong Healthy Aging Program, Centre for Molecular Medicine and Therapeutics, University of British Columbia, Vancouver, British Columbia, Canada. Department of Epidemiology, Columbia University Mailman School of Public Health, New York, NY, USA.
Center on Aging and Development, Biostatistics and Bioinformatics, Duke University, Durham, NC, USA. Duke Clinical Research Institute, Duke University School of Medicine, Durham, NC, USA.
Jean Mayer USDA Human Nutrition Research Center on Aging at Tufts University, Boston, MA, USA. Translational Gerontology Branch, National Institute on Aging, National Institutes of Health, Baltimore, MD, USA.
Department of Biobehavioral Health, Pennsylvania State University, State College, PA, USA. Pennington Biomedical Research Center, Baton Rouge, LA, USA.
Department of Medicine, Duke University School of Medicine, Durham, NC, USA. Program in Physical Therapy and Department of Medicine, Washington University School of Medicine, St. Louis, MO, USA. College of Health Solutions, Arizona State University, Phoenix, AZ, USA.
Buck Institute for Research on Aging, Novato, CA, USA. You can also search for this author in PubMed Google Scholar. designed the research. Kebbe, D. and B. conducted the research.
and D. prepared the DNA methylation datasets. analyzed the data. and R. wrote the first draft of the paper. wrote the revised draft of the paper. All authors contributed critical review of the paper. Correspondence to D. are listed as inventors on a Duke University and University of Otago invention, DunedinPACE, that was licensed to a commercial entity.
The other authors declare no competing interests. Nature Aging thanks the anonymous reviewers for their contribution to the peer review of this work.
Effects estimates of CR treatment from mixed models of change in epigenetic age used in Supplementary Fig. Effects estimates of CR treatment from mixed models of change in epigenetic age used in Fig. Open Access This article is licensed under a Creative Commons Attribution 4.
Reprints and permissions. Waziry, R. Effect of long-term caloric restriction on DNA methylation measures of biological aging in healthy adults from the CALERIE trial.
Nat Aging 3 , — Download citation. Received : 08 September Accepted : 22 December Published : 09 February Issue Date : March Anyone you share the following link with will be able to read this content:.
Sorry, a shareable link is not currently available for this article. Provided by the Springer Nature SharedIt content-sharing initiative. Sign up for the Nature Briefing newsletter — what matters in science, free to your inbox daily. Skip to main content Thank you for visiting nature.
nature nature aging letters article. Download PDF. Subjects Predictive markers. This article has been updated. Abstract The geroscience hypothesis proposes that therapy to slow or reverse molecular changes that occur with aging can delay or prevent multiple chronic diseases and extend healthy lifespan 1 , 2 , 3.
Main Comprehensive Assessment of Long-term Effects of Reducing Intake of Energy CALERIE Phase 2 was a multi-center, randomized controlled trial conducted at three clinical centers in the United States Full size image. Table 1 Characteristics of CALERIE Trial participants at baseline Full size table.
Table 2 DNAm clock and pace-of-aging measures included in CALERIE analysis Full size table. Methods We conducted new DNAm assays of stored blood biospecimens collected from the CALERIE Phase 2 randomized controlled trial and merged these data with existing secondary data from the trial.
Study design and participants CALERIE Phase 2 was a multi-center, randomized controlled trial conducted at three clinical centers in the United States 10 ClinicalTrials. Randomization and masking After baseline testing, participants were randomly assigned at a ratio of to a CR behavioral intervention or to an AL control group.
Procedures Study procedures were published previously 10 , 21 , 26 and are described here in brief. DNAm data DNA extracted from blood samples was obtained from the CALERIE Biorepository at the University of Vermont.
DNAm clocks and pace-of-aging measures DNAm clocks are algorithms that combine information from DNAm measurements across the genome to quantify variation in biological age Analysis Analysis included all participants with available DNAm data at trial baseline and at least one follow-up timepoint.
Specification of TOT regression models We tested TOT effects using two-stage least squares IV regression. References Kaeberlein, M. Article CAS PubMed PubMed Central Google Scholar Campisi, J.
Article CAS PubMed PubMed Central Google Scholar Speakman, J. Article CAS PubMed Google Scholar Maegawa, S. Article PubMed PubMed Central Google Scholar Hahn, O.
Article PubMed PubMed Central Google Scholar Petkovich, D. Article CAS PubMed PubMed Central Google Scholar Anderson, R. Article PubMed PubMed Central Google Scholar Mattison, J. Article CAS PubMed Google Scholar Ravussin, E. Article CAS Google Scholar Scott, A. Article PubMed PubMed Central Google Scholar Kaeberlein, M.
Article Google Scholar Goldman, D. Article Google Scholar Fahy, G. Article CAS PubMed PubMed Central Google Scholar Chen, L. Article CAS Google Scholar Sae-Lee, C. Article Google Scholar Colchero, F. Article CAS PubMed PubMed Central Google Scholar Fleming, T. Article PubMed PubMed Central Google Scholar Prentice, R.
Article CAS PubMed Google Scholar Justice, J. Article CAS PubMed PubMed Central Google Scholar Racette, S. Article CAS PubMed Google Scholar Levine, M.
Article PubMed PubMed Central Google Scholar Lu, A. Article CAS PubMed PubMed Central Google Scholar Belsky, D. Article CAS PubMed PubMed Central Google Scholar Higgins-Chen, A. Article PubMed PubMed Central Google Scholar Kraus, W. Article PubMed PubMed Central Google Scholar Benjamin, D.
Article PubMed Google Scholar Salas, L. Article PubMed PubMed Central Google Scholar Sugden, K. Article CAS PubMed PubMed Central Google Scholar Hillary, R. Article PubMed PubMed Central Google Scholar Belsky, D. Article Google Scholar Kwon, D.
Article CAS PubMed PubMed Central Google Scholar López-Otín, C. Article PubMed PubMed Central Google Scholar Spadaro, O.
Article CAS PubMed PubMed Central Google Scholar Redman, L. Article CAS PubMed PubMed Central Google Scholar Anthonisen, N. Article PubMed Google Scholar Ferrucci, L.
Article CAS PubMed Google Scholar Kritchevsky, S. Article Google Scholar Bell, C. PubMed Google Scholar Ahadi, S. Article CAS PubMed PubMed Central Google Scholar Ma, S. Article CAS PubMed Google Scholar Dorling, J. Article PubMed PubMed Central Google Scholar Das, S.
Article CAS PubMed PubMed Central Google Scholar Shen, W. Article PubMed PubMed Central Google Scholar Moffitt, T.
Article Google Scholar Sierra, F. Article PubMed PubMed Central Google Scholar Justice, J. Article PubMed PubMed Central Google Scholar Longo, V. Article PubMed PubMed Central Google Scholar Davis, S. Article CAS PubMed PubMed Central Google Scholar Lehne, B. Caloric restriction mimetics for the treatment of cardiovascular diseases.
Cardiovasc Res ; : — Bao Y , Ledderose C , Graf AF , Brix B , Birsak T , Lee A , Zhang J , Junger WG. mTOR and differential activation of mitochondria orchestrate neutrophil chemotaxis.
J Cell Biol ; : — Vitiello D , Neagoe PE , Sirois MG , White M. Effect of everolimus on the immunomodulation of the human neutrophil inflammatory response and activation. Cell Mol Immunol ; 12 : 40 — Porsch F , Mallat Z , Binder CJ.
Humoral immunity in atherosclerosis and myocardial infarction: from B cells to antibodies. Nath N , Khan M , Paintlia MK , Singh I , Hoda MN , Giri S. Metformin attenuated the autoimmune disease of the central nervous system in animal models of multiple sclerosis. Metformin ameliorates the development of experimental autoimmune encephalomyelitis by regulating T helper 17 and regulatory T cells in mice.
J Neuroimmunol ; : 58 — Negrotto L , Farez MF , Correale J. Immunologic effects of metformin and pioglitazone treatment on metabolic syndrome and multiple sclerosis.
JAMA Neurol ; 73 : — Bharath LP , Agrawal M , McCambridge G , Nicholas DA , Hasturk H , Liu J , Jiang K , Liu R , Guo Z , Deeney J , Apovian CM , Snyder-Cappione J , Hawk GS , Fleeman RM , Pihl RMF , Thompson K , Belkina AC , Cui L , Proctor EA , Kern PA , Nikolajczyk BS.
Metformin enhances autophagy and normalizes mitochondrial function to alleviate aging-associated inflammation.
Cell Metab ; 32 : 44 — Cipolletta D , Feuerer M , Li A , Kamei N , Lee J , Shoelson SE , Benoist C , Mathis D. PPAR-γ is a major driver of the accumulation and phenotype of adipose tissue Treg cells. Nature ; : — Simental-Mendía LE , Pirro M , Gotto AM Jr , Banach M , Atkin SL , Majeed M , Sahebkar A.
Lipid-modifying activity of curcuminoids: a systematic review and meta-analysis of randomized controlled trials. Crit Rev Food Sci Nutr ; 59 : — Tabrizi R , Tamtaji OR , Mirhosseini N , Lankarani KB , Akbari M , Heydari ST , Dadgostar E , Asemi Z. The effects of quercetin supplementation on lipid profiles and inflammatory markers among patients with metabolic syndrome and related disorders: a systematic review and meta-analysis of randomized controlled trials.
Crit Rev Food Sci Nutr ; 60 : — Abdellatif M , Trummer-Herbst V , Koser F , Durand S , Adão R , Vasques-Nóvoa F , Freundt JK , Voglhuber J , Pricolo MR , Kasa M , Türk C , Aprahamian F , Herrero-Galán E , Hofer SJ , Pendl T , Rech L , Kargl J , Anto-Michel N , Ljubojevic-Holzer S , Schipke J , Brandenberger C , Auer M , Schreiber R , Koyani CN , Heinemann A , Zirlik A , Schmidt A , von Lewinski D , Scherr D , Rainer PP , von Maltzahn J , Mühlfeld C , Krüger M , Frank S , Madeo F , Eisenberg T , Prokesch A , Leite-Moreira AF , Lourenço AP , Alegre-Cebollada J , Kiechl S , Linke WA , Kroemer G , Sedej S.
Nicotinamide for the treatment of heart failure with preserved ejection fraction. Sci Transl Med ; 13 : eabd Wan R , Camandola S , Mattson MP. Intermittent fasting and dietary supplementation with 2-deoxy-D-glucose improve functional and metabolic cardiovascular risk factors in rats. FASEB J ; 17 : — Hawley SA , Fullerton MD , Ross FA , Schertzer JD , Chevtzoff C , Walker KJ , Peggie MW , Zibrova D , Green KA , Mustard KJ , Kemp BE , Sakamoto K , Steinberg GR , Hardie DG.
The ancient drug salicylate directly activates AMP-activated protein kinase. Mol Med Rep ; 15 : — Stumvoll M , Nurjhan N , Perriello G , Dailey G , Gerich JE.
Metabolic effects of metformin in non-insulin-dependent diabetes mellitus. N Engl J Med ; : — Madeo F , Carmona-Gutierrez D , Hofer SJ , Kroemer G. Caloric restriction mimetics against age-associated disease: targets, mechanisms, and therapeutic potential.
Cell Metab ; 29 : — Katsyuba E , Romani M , Hofer D , Auwerx J. Nat Metab ; 2 : 9 — Hotamisligil GS. Inflammation, metaflammation and immunometabolic disorders. Lips MA , van Klinken JB , Pijl H , Janssen I , van Dijk K W , Koning F , van Harmelen V.
Weight loss induced by very low calorie diet is associated with a more beneficial systemic inflammatory profile than by Roux-en-Y gastric bypass. Metabolism ; 65 : — Cantoni C , Dorsett Y , Fontana L , Zhou Y , Piccio L. Effects of dietary restriction on gut microbiota and CNS autoimmunity. Clin Immunol ; : Li Z , Bowers E , Zhu J , Yu H , Hardij J , Bagchi DP , Mori H , Lewis KT , Granger K , Schill RL , Romanelli SM , Abrishami S , Hankenson KD , Singer K , Rosen CJ , MacDougald OA.
Lipolysis of bone marrow adipocytes is required to fuel bone and the marrow niche during energy deficits. Elife ; 11 : e Takakuwa T , Nakashima Y , Koh H , Nakane T , Nakamae H , Hino M. Short-term fasting induces cell cycle arrest in immature hematopoietic cells and increases the number of naïve T cells in the bone marrow of mice.
Acta Haematol ; : — Contreras NA , Fontana L , Tosti V , Nikolich-Zugich J. Calorie restriction induces reversible lymphopenia and lymphoid organ atrophy due to cell redistribution. Geroscience ; 40 : — Lazare S , Ausema A , Reijne AC , van Dijk G , van Os R , de Haan G. Lifelong dietary intervention does not affect hematopoietic stem cell function.
Exp Hematol ; 53 : 26 — Goldberg EL , Dixit VD. Bone marrow: an immunometabolic refuge during energy depletion. Cell Metab ; 30 : — Mazo IB , Honczarenko M , Leung H , Cavanagh LL , Bonasio R , Weninger W , Engelke K , Xia L , McEver RP , Koni PA , Silberstein LE , von Andrian UH.
Immunity ; 22 : — Aoki K , Kurashige M , Ichii M , Higaki K , Sugiyama T , Kaito T , Ando W , Sugano N , Sakai T , Shibayama H , Takaori-Kondo A , Morii E , Kanakura Y , Nagasawa T ; HANDAI Clinical Blood Club.
Identification of CXCLabundant reticular cells in human adult bone marrow. Br J Haematol ; : — Sugiyama T , Kohara H , Noda M , Nagasawa T.
Maintenance of the hematopoietic stem cell pool by CXCLCXCR4 chemokine signaling in bone marrow stromal cell niches. Immunity ; 25 : — Besedovsky L , Linz B , Born J , Lange T.
Mineralocorticoid receptor signaling reduces numbers of circulating human naïve T cells and increases their CD62L, CCR7, and CXCR4 expression. Eur J Immunol ; 44 : — Shimba A , Cui G , Tani-Ichi S , Ogawa M , Abe S , Okazaki F , Kitano S , Miyachi H , Yamada H , Hara T , Yoshikai Y , Nagasawa T , Schütz G , Ikuta K.
Glucocorticoids drive diurnal oscillations in T cell distribution and responses by inducing interleukin-7 receptor and CXCR4. Immunity ; 48 : — Fujita Y , Murakami M , Ogawa Y , Masuzaki H , Tanaka M , Ozaki S , Nakao K , Mimori T.
Leptin inhibits stress-induced apoptosis of T lymphocytes. Clin Exp Immunol ; : 21 — Wing EJ , Magee DM , Barczynski LK. Acute starvation in mice reduces the number of T cells and suppresses the development of T-cell-mediated immunity.
Immunology ; 63 : — Serbina NV , Pamer EG. Monocyte emigration from bone marrow during bacterial infection requires signals mediated by chemokine receptor CCR2. Nature immunology ; 7 : — Shi C , Jia T , Mendez-Ferrer S , Hohl TM , Serbina NV , Lipuma L , Leiner I , Li MO , Frenette PS , Pamer EG.
Bone marrow mesenchymal stem and progenitor cells induce monocyte emigration in response to circulating toll-like receptor ligands. Immunity ; 34 : — Pålsson-McDermott EM , O'Neill LAJ. Targeting immunometabolism as an anti-inflammatory strategy. Cell Res ; 30 : — Michalek RD , Gerriets VA , Jacobs SR , Macintyre AN , MacIver NJ , Mason EF , Sullivan SA , Nichols AG , Rathmell JC.
Pearce EL , Walsh MC , Cejas PJ , Harms GM , Shen H , Wang LS , Jones RG , Choi Y. Enhancing CD8 T-cell memory by modulating fatty acid metabolism. Bruss MD , Khambatta CF , Ruby MA , Aggarwal I , Hellerstein MK. Calorie restriction increases fatty acid synthesis and whole body fat oxidation rates.
Berod L , Friedrich Y , Nandan A , Freitag J , Hagemann S , Harmrolfs K , Sandouk A , Hesse C , Castro CN , Bähre H , Tschirner SK , Gorinski N , Gohmert M , Mayer CT , Huehn J , Ponimaskin E , Abraham WR , Müller R , Lochner M , Sparwasser T.
De novo fatty acid synthesis controls the fate between regulatory T and T helper 17 cells. Nat Med ; 20 : — Endo Y , Onodera A , Obata-Ninomiya K , Koyama-Nasu R , Asou HK , Ito T , Yamamoto T , Kanno T , Nakajima T , Ishiwata K , Kanuka H , Tumes DJ , Nakayama T.
Nat Metab ; 1 : — Int Immunopharmacol ; 39 : — Yang H , Yang T , Baur JA , Perez E , Matsui T , Carmona JJ , Lamming DW , Souza-Pinto NC , Bohr VA , Rosenzweig A , de Cabo R , Sauve AA , Sinclair DA.
Lin SJ , Defossez PA , Guarente L. Requirement of NAD and SIR2 for life-span extension by calorie restriction in Saccharomyces cerevisiae. Cantó C , Gerhart-Hines Z , Feige JN , Lagouge M , Noriega L , Milne JC , Elliott PJ , Puigserver P , Auwerx J.
SIRT1 and HIF1a signaling in metabolism and immune responses. Cancer Lett ; : 20 — Molecular cell sirtuin 1 modulates cellular responses to hypoxia by deacetylating hypoxia-inducible factor 1a.
Mol Cell ; 38 : — Bekkering S , Joosten LA , van der Meer JW , Netea MG , Riksen NP. Trained innate immunity and atherosclerosis. Curr Opin Lipidol ; 24 : — Diskin C , Pålsson-McDermott EM. Metabolic modulation in macrophage effector function. Front Immunol ; 9 : Hudson CC , Liu M , Chiang GG , Otterness DM , Loomis DC , Kaper F , Giaccia AJ , Abraham RT.
Regulation of hypoxia-inducible factor 1alpha expression and function by the mammalian target of rapamycin. Mol Cell Biol ; 22 : — Cheng SC , Quintin J , Cramer RA , Shepardson KM , Saeed S , Kumar V , Giamarellos-Bourboulis EJ , Martens JH , Rao NA , Aghajanirefah A , Manjeri GR , Li Y , Ifrim DC , Arts RJ , van der Veer BM , Deen PM , Logie C , O'Neill LA , Willems P , van de Veerdonk FL , van der Meer JW , Ng A , Joosten LA , Wijmenga C , Stunnenberg HG , Xavier RJ , Netea MG.
mTOR- and HIF-1α-mediated aerobic glycolysis as metabolic basis for trained immunity. Science ; : Lee YH , Petkova AP , Granneman JG. Identification of an adipogenic niche for adipose tissue remodeling and restoration. Cell Metab ; 18 : — Wernstedt Asterholm I , Tao C , Morley TS , Wang QA , Delgado-Lopez F , Wang ZV , Scherer PE.
Adipocyte inflammation is essential for healthy adipose tissue expansion and remodeling. Cell Metab ; 20 : — Foundations of immunometabolism and implications for metabolic health and disease. Immunity ; 47 : — Spadaro O , Youm Y , Shchukina I , Ryu S , Sidorov S , Ravussin A , Nguyen K , Aladyeva E , Predeus AN , Smith SR , Ravussin E , Galban C , Artyomov MN , Dixit VD.
Caloric restriction in humans reveals immunometabolic regulators of health span. Lee AH , Dixit VD. Dietary regulation of immunity. Immunity ; 53 : — Fabbiano S , Suárez-Zamorano N , Rigo D , Veyrat-Durebex C , Stevanovic Dokic A , Colin DJ , Trajkovski M.
Caloric restriction leads to browning of white adipose tissue through type 2 immune signaling. Cell Metab ; 24 : — Intermittent fasting promotes adipose thermogenesis and metabolic homeostasis via VEGF-mediated alternative activation of macrophage. Cell Res ; 27 : — Odegaard JI , Chawla A.
Type 2 responses at the interface between immunity and fat metabolism. Curr Opin Immunol ; 36 : 67 — Dixit VD. Thymic fatness and approaches to enhance thymopoietic fitness in aging.
Curr Opin Immunol ; 22 : — Stein S , Lohmann C , Schäfer N , Hofmann J , Rohrer L , Besler C , Rothgiesser KM , Becher B , Hottiger MO , Borén J , McBurney MW , Landmesser U , Lüscher TF , Matter CM.
SIRT1 decreases Loxmediated foam cell formation in atherogenesis. Eur Heart J ; 31 : — Winnik S , Stein S , Matter CM.
SIRT1—an anti-inflammatory pathway at the crossroads between metabolic disease and atherosclerosis. Curr Vasc Pharmacol ; 10 : — Bartelt A , Heeren J.
Adipose tissue browning and metabolic health. Nat Rev Endocrinol ; 10 : 24 — Kosteli A , Sugaru E , Haemmerle G , Martin JF , Lei J , Zechner R , Ferrante AW Jr. Weight loss and lipolysis promote a dynamic immune response in murine adipose tissue.
Wensveen FM , Valentic S , Sestan M , Wensveen TT , Polic B. Interactions between adipose tissue and the immune system in health and malnutrition. Semin Immunol ; 27 : — Wu H , Ghosh S , Perrard XD , Feng L , Garcia GE , Perrard JL , Sweeney JF , Peterson LE , Chan L , Smith CW , Ballantyne CM.
T-cell accumulation and regulated on activation, normal T cell expressed and secreted upregulation in adipose tissue in obesity. Rocha VZ , Libby P. Obesity, inflammation, and atherosclerosis.
Nat Rev Cardiol ; 6 : — Huber J , Kiefer FW , Zeyda M , Ludvik B , Silberhumer GR , Prager G , Zlabinger GJ , Stulnig TM.
CC Chemokine and CC chemokine receptor profiles in visceral and subcutaneous adipose tissue are altered in human obesity. J Clin Endocrinol Metab ; 93 : — Khan IM , Dai Perrard XY , Perrard JL , Mansoori A , Smith CW , Wu H , Ballantyne CM.
Atherosclerosis ; : — Deng T , Lyon CJ , Minze LJ , Lin J , Zou J , Liu JZ , Ren Y , Yin Z , Hamilton DJ , Reardon PR , Sherman V , Wang HY , Phillips KJ , Webb P , Wong ST , Wang RF , Hsueh WA.
Class II major histocompatibility complex plays an essential role in obesity-induced adipose inflammation. Cell Metab ; 17 : — Cell Rep ; 9 : — Morris DL , Cho KW , Delproposto JL , Oatmen KE , Geletka LM , Martinez-Santibanez G , Singer K , Lumeng CN.
Diabetes ; 62 : — Khan IM , Perrard XY , Brunner G , Lui H , Sparks LM , Smith SR , Wang X , Shi ZZ , Lewis DE , Wu H , Ballantyne CM. Intermuscular and perimuscular fat expansion in obesity correlates with skeletal muscle T cell and macrophage infiltration and insulin resistance.
Int J Obes ; 39 : — Nishimura S , Manabe I , Nagasaki M , Eto K , Yamashita H , Ohsugi M , Otsu M , Hara K , Ueki K , Sugiura S , Yoshimura K , Kadowaki T , Nagai R. Nat Med ; 15 : — Feuerer M , Herrero L , Cipolletta D , Naaz A , Wong J , Nayer A , Lee J , Goldfine AB , Benoist C , Shoelson S , Mathis D.
Lean, but not obese, fat is enriched for a unique population of regulatory T cells that affect metabolic parameters. Panduro M , Benoist C , Mathis D.
Tissue Tregs. Annu Rev Immunol ; 34 : — Yan X , Imano N , Tamaki K , Sano M , Shinmura K. The effect of caloric restriction on the increase in senescence-associated T cells and metabolic disorders in aged mice.
PLoS One ; 16 : e Time-restricted feeding restores obesity-induced alteration in adipose tissue immune cell phenotype. Stampanoni Bassi M , Iezzi E , Buttari F , Gilio L , Simonelli I , Carbone F , Micillo T , De Rosa V , Sica F , Furlan R , Finardi A , Fantozzi R , Storto M , Bellantonio P , Pirollo P , Di Lemme S , Musella A , Mandolesi G , Centonze D , Matarese G.
Obesity worsens central inflammation and disability in multiple sclerosis. Mult Scler ; 26 : — Mouton AJ , Li X , Hall ME , Hall JE. Obesity, hypertension, and cardiac dysfunction: novel roles of immunometabolism in macrophage activation and inflammation.
Procaccini C , Carbone F , Di Silvestre D , Brambilla F , De Rosa V , Galgani M , Faicchia D , Marone G , Tramontano D , Corona M , Alviggi C , Porcellini A , La Cava A , Mauri P , Matarese G. The proteomic landscape of human ex vivo regulatory and conventional T cells reveals specific metabolic requirements.
Immunity ; 44 : Procaccini C , De Rosa V , Galgani M , Abanni L , Calì G , Porcellini A , Carbone F , Fontana S , Horvath TL , La Cava A , Matarese G. An oscillatory switch in mTOR kinase activity sets regulatory T cell responsiveness. Immunity ; 33 : — Procaccini C , De Rosa V , Galgani M , Carbone F , Cassano S , Greco D , Qian K , Auvinen P , Calì G , Stallone G , Formisano L , La Cava A , Matarese G.
Matarese G , La Cava A , Horvath TL. In vivo veritas, in vitro artificia. Trends Mol Med ; 18 : — Procaccini C , Garavelli S , Carbone F , Di Silvestre D , La Rocca C , Greco D , Colamatteo A , Lepore MT , Russo C , De Rosa G , Faicchia D , Prattichizzo F , Grossi S , Campomenosi P , Buttari F , Mauri P , Uccelli A , Salvetti M , Brescia Morra V , Vella D , Galgani M , Mottola M , Zuccarelli B , Lanzillo R , Maniscalco GT , Centonze D , de Candia P , Matarese G.
Signals of pseudo-starvation unveil the amino acid transporter SLC7A11 as key determinant in the control of Treg cell proliferative potential. Immunity ; 54 : — De Rosa V , Procaccini C , La Cava A , Chieffi P , Nicoletti GF , Fontana S , Zappacosta S , Matarese G.
Leptin neutralization interferes with pathogenic T cell autoreactivity in autoimmune encephalomyelitis. J Neuroimmunol ; : 26 — Modulation of the immune response in rheumatoid arthritis with strategically released rapamycin.
Mol Med Rep ; 16 : — De Rosa V , Procaccini C , Calì G , Pirozzi G , Fontana S , Zappacosta S , La Cava A , Matarese G. A key role of leptin in the control of regulatory T cell proliferation. Immunity ; 26 : — Kristófi R , Eriksson JW. Metformin as an anti-inflammatory agent: a short review.
J Endocrinol ; : R11 — R Br J Dermatol ; : — Mariño G , Pietrocola F , Madeo F , Kroemer G. Autophagy ; 10 : — Becher J , Simula L , Volpe E , Procaccini C , La Rocca C , D'Acunzo P , Cianfanelli V , Strappazzon F , Caruana I , Nazio F , Weber G , Gigantino V , Botti G , Ciccosanti F , Borsellino G , Campello S , Mandolesi G , De Bardi M , Fimia GM , D'Amelio M , Ruffini F , Furlan R , Centonze D , Martino G , Braghetta P , Chrisam M , Bonaldo P , Matarese G , Locatelli F , Battistini L , Cecconi F.
AMBRA1 controls regulatory T-cell differentiation and homeostasis upstream of the FOXO3-FOXP3 axis. Dev Cell ; 47 : — Cassano S , Pucino V , La Rocca C , Procaccini C , De Rosa V , Marone G , Matarese G.
Metabolism ; 63 : — Pietrocola F , Pol J , Vacchelli E , Rao S , Enot DP , Baracco EE , Levesque S , Castoldi F , Jacquelot N , Yamazaki T , Senovilla L , Marino G , Aranda F , Durand S , Sica V , Chery A , Lachkar S , Sigl V , Bloy N , Buque A , Falzoni S , Ryffel B , Apetoh L , Di Virgilio F , Madeo F , Maiuri MC , Zitvogel L , Levine B , Penninger JM , Kroemer G.
Caloric restriction mimetics enhance anticancer immunosurveillance. Cancer Cell ; 30 : — Mizushima N , Yamamoto A , Matsui M , Yoshimori T , Ohsumi Y. to a 25 percent calorie-restriction or normal diet for two years.
Instead, we rely on biomarkers developed to measure the pace and progress of biological aging over the duration of the study. DNA methylation marks are chemical tags on the DNA sequence that regulate the expression of genes and are known to change with aging. The third measure studied by the researchers was DunedinPACE, which estimates the pace of aging, or the rate of biological deterioration over time.
Our findings are important because they provide evidence from a randomized trial that slowing human aging may be possible. They also give us a sense of the kinds of effects we might look for in trials of interventions that could appeal to more people, like intermittent fasting or time-restricted eating.
In other studies, slower DunedinPACE is associated with reduced risk for heart disease, stroke, disability, and dementia. DunedinPACE was developed by Daniel Belsky and colleagues at Duke University and the University of Otago.
Laboratory studies of animals, including rats, fruit cqloric, worms, and mice, caloric restriction and cellular health that those daloric a calorie-restricted diet may live up celllular twice Optimize personal relationships long as Optimize personal relationships restritcion an unrestricted diet. Now, a team restrictlon by researchers from Yale University has investigated the effects of calorie restriction in people. Their findings, which appear in Sciencemay eventually lead to new ways to extend healthy life. However, as the authors of the new study explain, this effects growth, reproduction, and immunity. Unlike many weight loss diets, a calorie-restricted diet involves small reductions of habitual calorie intake over a long period. People usually lose some weight, but this is not the main aim of calorie restriction.Caloric restriction and cellular health -
They also give us a sense of the kinds of effects we might look for in trials of interventions that could appeal to more people, like intermittent fasting or time-restricted eating. In other studies, slower DunedinPACE is associated with reduced risk for heart disease, stroke, disability, and dementia.
DunedinPACE was developed by Daniel Belsky and colleagues at Duke University and the University of Otago. To develop DunedinPACE, researchers analyzed data from the Dunedin Longitudinal Study, a landmark birth cohort study of human development and aging that follows individuals born in in Dunedin, New Zealand.
Researchers first analyzed the rate of change in 19 biomarkers across 20 years of follow-up to derive a single composite measure of the Pace of Aging. Next, the researchers used machine-learning techniques to distill this year Pace of Aging into a single-time-point DNA methylation blood test.
The values of the DunedinPACE algorithm correspond to the years of biological aging experienced during a single calendar year, providing a measure of the pace of aging.
Additional co-authors and their affiliations are listed in the paper, "Effect of long-term caloric restriction on DNA methylation measures of biological aging in healthy adults from the CALERIE trial. Our findings highlight DunedinPACE as a measure with potential utility in future trials.
DunedinPACE has high test—retest reliability and shows strong associations with healthspan endpoints in validation analyses 24 , Ultimately, establishing DunedinPACE and other DNAm measures of aging as surrogate endpoints for geroscience will require evidence that changes in DNAm measures account for intervention effects on primary healthy-aging endpoints, including incidence of chronic disease and mortality 18 , 19 , The evidence reported from CALERIE suggests that DunedinPACE may be helpful in identifying short-term interventions worthy of long-term follow-up to generate such evidence.
CALERIE was a month, intensive behavioral intervention to deliver a therapy proven to slow aging in animal models. Although treatment effect sizes were small, even modest slowing of the pace of aging can have profound effects on population health 11 , 12 , Future trials, especially those considering less-intensive or shorter-term interventions, such as intermittent fasting 50 , should plan for larger samples to ensure adequate statistical power.
Further, efforts to forecast potential benefits from interventions designed to delay aging may best serve policy makers and planners if they work from assumptions of modest intervention effects.
We conducted new DNAm assays of stored blood biospecimens collected from the CALERIE Phase 2 randomized controlled trial and merged these data with existing secondary data from the trial. The assays of the biospecimens were conducted blind to the conditions of the trial.
Details of trial design and the collection of other trial data were reported previously 10 , CALERIE Phase 2 was a multi-center, randomized controlled trial conducted at three clinical centers in the United States 10 ClinicalTrials.
gov Identifier: NCT The study protocol was approved by Institutional Review Boards at three clinical centers Washington University School of Medicine, St Louis, MO, USA; Pennington Biomedical Research Center, Baton Rouge, LA, USA; Tufts University, Boston, MA, USA and the coordinating center at Duke University Durham, NC, USA.
All study participants provided written, informed consent. After baseline testing, participants were randomly assigned at a ratio of to a CR behavioral intervention or to an AL control group.
Randomization was stratified by site, sex and BMI. A permuted block randomization technique was used. Study procedures were published previously 10 , 21 , 26 and are described here in brief.
Participants also received instruction on the essentials of CR. Adherence to the CR intervention was estimated in real time by the degree to which individual weight change followed a predicted weight loss trajectory The precise level of CR achieved was quantified retrospectively by calculating energy intake during the CR intervention and comparing it with baseline energy intake.
Participants assigned to the AL group continued on their regular diets; they received no specific dietary intervention or counseling. They had quarterly contact with study investigators to complete the assessments. TEE was measured by the DLW method during two consecutive 2-week periods at baseline and during 2-week periods at months 6, 12, 18 and 24 in the CR group 10 , DNA extracted from blood samples was obtained from the CALERIE Biorepository at the University of Vermont.
DNAm data were generated by the Kobor Lab at the University of British Columbia and processed by the Genomic Analysis and Bioinformatics Shared Resource at Duke University. Illumina Infinium Methylation EPIC BeadChip arrays were used to assay genome-wide DNAm data from banked DNA samples extracted from blood collected at the baseline, month and month follow-ups.
To the extent possible, baseline, month and month samples from the same individual were processed in the same array batch and on the same BeadChip to minimize batch effects; CR treatment and AL control participants were included on all chips. Quality control and normalization analyses were performed using the methylumi v.
Normalization to eliminate systematic dye bias in 2-channel probes was carried out using the methylumi default method. Additional batch correction was performed by residualizing DNAm measurements for PCs estimated from array control-probe beta values Cell count estimation was performed using the Houseman equation via the minfi and FlowSorted.
EPIC R packages 28 , DNAm clocks are algorithms that combine information from DNAm measurements across the genome to quantify variation in biological age The first-generation DNAm clocks were developed from machine-learning analyses comparing samples from individuals of different chronological age.
These clocks were highly accurate in predicting the chronological age of new samples and also showed some capacity for predicting differences in mortality risk, although effect sizes tend to be small and inconsistent across studies 56 , 57 , We analyzed the first-generation clocks proposed by Horvath Horvath clock and Hannum et al.
Hannum clock 56 , The second-generation DNAm clocks were developed with the goal of improving quantification of biological aging by focusing on differences in mortality risk instead of on differences in chronological age 22 , These clocks also include an intermediate step in which DNAm data are fitted to physiological parameters.
The second-generation clocks are more predictive of morbidity and mortality as compared with the first-generation clocks 59 and are proposed to have improved potential for testing impacts of interventions to slow aging We analyzed the second-generation clocks proposed by Levine et al.
PhenoAge clock and Lu et al. GrimAge clock 22 , A limitation of several DNAm clocks is that when residualized for chronological age, values show only moderate test—retest reliability across technical replicates. Test—retest reliability is a critical feature of measurements used to evaluate the impact of intervention because change from preintervention to postintervention cannot be distinguished from technical noise unless reliability is high.
To improve technical reliability, Higgins-Chen and colleagues developed a new computational method that retrained DNAm clocks using DNAm PCs A third generation of DNAm measures of aging are referred to as pace-of-aging measures.
In contrast to first- and second-generation DNAm clocks, which aim to quantify how much aging has occurred up to the time of measurement, pace-of-aging measures aim to quantity how fast the process of aging-related deterioration of system integrity is proceeding.
Slopes of change were estimated from four repeated measurements collected over a period of two decades. This physiological pace-of-aging composite is described in detail in ref.
The DunedinPACE DNAm algorithm was derived from elastic net regression of the physiological pace-of-aging composite on Illumina EPIC array DNAm data derived from blood samples collected at the age 45 follow-up assessment.
The set of CpG sites included in the DNAm dataset used to develop the DunedinPACE algorithm was restricted to those showing acceptable test—retest reliability as determined in the analysis in ref.
The DunedinPACE DNAm algorithm is described in detail in ref. Our primary analysis focused on the PC versions of the PhenoAge and GrimAge second-generation clocks and DunedinPACE, all of which show exceptional test—retest reliability in technical replicates. We report results for both original and PC versions of DNAm clocks in the Supplementary Information.
Analysis included all participants with available DNAm data at trial baseline and at least one follow-up timepoint. We conducted analyses of these change scores to test the hypothesis that CR slows biological aging using two complementary approaches: 1 we conducted ITT analysis which compared change scores between participants randomized to CR intervention and the AL control group; 2 we conducted TOT analysis using IV methods to estimate the effect of CR on change scores.
In ITT analysis, we tested the effect of randomization to CR versus AL on aging measure change scores using repeated-measures ANCOVA implemented under mixed models, following the approach used in past CALERIE analysis In TOT analysis, we tested the effect of the CR intervention on aging measure change scores using IV regression implemented using a two-stage least squares approach The model instruments were randomization condition and interactions of randomization condition with sex and pretreatment values of BMI and the biological aging measure.
The second-stage regression modeled aging measure change scores as a function of the CR treatment dose estimated from the first-stage regression and pretreatment covariates. Separate models were fitted for the and month follow-ups.
IV regression models were fitted using the Stata TOT models are described in detail below. In ITT and TOT analyses, effect sizes were scaled in standardized units according to the distribution of the aging measures at pretreatment baseline. For the DNAm clocks, clock ages were differenced from chronological ages and standard deviations for these age-difference values were used for scaling.
For DunedinPACE, the standard deviations of the original values were used for scaling. We tested TOT effects using two-stage least squares IV regression. IV regression is a method commonly used to reduce the impact of confounding in association analysis.
Under conditions of nonadherence, traditional ITT analysis can result in a biased estimate of the treatment effect and an IV estimator can provide a complement The ITT estimate may therefore underestimate the effect of CR on biological aging. The IV approach we used involved two related regressions.
The second regression modeled the outcomes changes in measures of biological aging as functions of the predicted treatment dose estimated by the first regression and pretreatment covariates.
The base first-stage regression took the form. Results from this first-stage regression were then included in the second-stage model:. For final TOT analysis, we included a further instrument in the first-stage regression consisting of the interaction between the baseline level of the aging measure and the CR treatment group.
Sensitivity analysis involving re-estimating the IV regression models omitting this final instrument did not change results. Supplementary Fig. Data met model assumptions. Normality of outcome variables was evaluated by visual inspection of distributions and the Shapiro—Wilk test Equality of variances was evaluated according to the tests proposed by Brown and Forsythe 68 and Markowski and Markowski Models used to test ITT and TOT effects were fitted with heteroskedasticity-robust standard errors.
Normality of distribution of error terms was evaluated by visual inspection of histograms of residuals and the Shapiro—Wilk test. The clocks we analyzed were developed to predict mortality risk. The age values computed by the clock algorithms correspond to the age at which predicted mortality risk would be approximately normal in the reference population used to develop the clock.
Pace-of-aging measures estimate the rate of biological aging, defined as the rate of decline in overall system integrity. Pace-of-aging values correspond to the years of biological aging experienced during a single calendar year.
A value of 1 represents the typical pace of aging in a reference population; values above 1 indicate faster pace of aging; values below 1 indicate slower pace of aging. Further information on research design is available in the Nature Portfolio Reporting Summary linked to this article.
Applications for some types of data may require IRB oversight. Source data for Fig. Kaeberlein, M. Longevity and aging. FPrime Rep. Kennedy, B. et al. Geroscience: linking aging to chronic disease. Cell , — Article CAS PubMed PubMed Central Google Scholar.
Campisi, J. From discoveries in ageing research to therapeutics for healthy ageing. Nature , — Speakman, J. Caloric restriction. Aspects Med. Article CAS PubMed Google Scholar. Maegawa, S. Caloric restriction delays age-related methylation drift. Article PubMed PubMed Central Google Scholar.
Hahn, O. Dietary restriction protects from age-associated DNA methylation and induces epigenetic reprogramming of lipid metabolism. Genome Biol. Petkovich, D. Using DNA methylation profiling to evaluate biological age and longevity interventions.
Cell Metab. e6 Anderson, R. The caloric restriction paradigm: implications for healthy human aging. Mattison, J. Impact of caloric restriction on health and survival in rhesus monkeys from the NIA study. Ravussin, E. A 2-year randomized controlled trial of human caloric restriction: feasibility and effects on predictors of health span and longevity.
A 70 , — Article CAS Google Scholar. Scott, A. The economic value of targeting aging. Aging 1 , — It is time to embrace 21st-century medicine. Public Policy Aging Rep. Article Google Scholar. Goldman, D. Substantial health and economic returns from delayed aging may warrant a new focus for medical research.
Health Aff. Fahy, G. Reversal of epigenetic aging and immunosenescent trends in humans. Aging Cell 18 , e Chen, L. Effects of vitamin D3 supplementation on epigenetic aging in overweight and obese African Americans with suboptimal vitamin D status: a randomized clinical trial.
A 74 , 91—98 Sae-Lee, C. Dietary intervention modifies DNA methylation age assessed by the epigenetic clock. Food Res. Colchero, F. Fleming, T. Biomarkers and surrogate endpoints in clinical trials. Prentice, R. Surrogate endpoints in clinical trials: definition and operational criteria.
Justice, J. A framework for selection of blood-based biomarkers for geroscience-guided clinical trials: report from the TAME Biomarkers Workgroup.
GeroScience 40 , — Racette, S. Levine, M. An epigenetic biomarker of aging for lifespan and healthspan. Aging 10 , — Lu, A. DNA methylation GrimAge strongly predicts lifespan and healthspan. Aging 11 , — Belsky, D.
DunedinPACE, a DNA methylation biomarker of the pace of aging. eLife 11 , e Higgins-Chen, A. A computational solution for bolstering reliability of epigenetic clocks: implications for clinical trials and longitudinal tracking.
Aging 2 , — Kraus, W. Lancet Diabetes Endocrinol. Benjamin, D. Redefine statistical significance. Article PubMed Google Scholar. Salas, L. An optimized library for reference-based deconvolution of whole-blood biospecimens assayed using the Illumina HumanMethylationEPIC BeadArray.
Sugden, K. Association of pace of aging measured by blood-based DNA methylation with age-related cognitive impairment and dementia.
Neurology 99 , e—e Hillary, R. Epigenetic measures of ageing predict the prevalence and incidence of leading causes of death and disease burden. Epigenetics 12 , Change in the rate of biological aging in response to caloric restriction: CALERIE Biobank Analysis. A 73 , 4—10 Kwon, D. A toolkit for quantification of biological age from blood chemistry and organ function test data: BioAge.
Geroscience 43 , — López-Otín, C. The hallmarks of aging. Spadaro, O. Caloric restriction in humans reveals immunometabolic regulators of health span. Science , — Redman, L. Metabolic slowing and reduced oxidative damage with sustained caloric restriction support the rate of living and oxidative damage theories of aging.
How caloric restriction prevents negative effects of aging in cells. Retrieved February 14, from www. htm accessed February 14, Explore More.
Calorie Restriction in Humans Builds Strong Muscle and Stimulates Healthy Aging Genes. Decreasing calories without depriving the body of Cutting Calories and Eating at the Right Time of Day Leads to Longer Life in Mice.
May 5, In a study that followed hundreds of mice over their lifespans, calorie restriction combined with time-restricted eating boosted Fasting Is Required to See the Full Benefit of Calorie Restriction in Mice.
Researchers have largely assumed that reduced food intake drove Eat Less and Live a Long Healthy Life? Study Shows 'Not in All Cases'. June 4, The assumption that dietary restriction and drugs that mimic its effects will extend both lifespan and healthspan jointly has come under question, based on research involving genetically Print Email Share.
Trending Topics. Breast Cancer. Personalized Medicine. Child Development. Consumer Behavior. Smart Earrings Can Monitor a Person's Temperature. Researchers 3D-Print Functional Human Brain Tissue.
A Long-Lasting Neural Probe. Great Apes Playfully Tease Each Other. How Teachers Make Ethical Judgments When Using AI in the Classroom.
Caloric restriction and cellular health you for visiting nature. Restroction are using a hhealth version with limited support for Cakoric. To obtain healgh best experience, we recommend you use caloric restriction and cellular health more Sodium-free diet to date browser or turn off compatibility mode in Internet Explorer. Enhance insulin signaling the restiction, to ensure continued support, we are displaying the site without styles and JavaScript. Dietary restriction with adequate nutrition is the gold standard for delaying ageing and extending healthspan and lifespan in diverse species, including rodents and non-human primates. In this Review, we discuss the effects of dietary restriction in these mammalian model organisms and discuss accumulating data that suggest that dietary restriction results in many of the same physiological, metabolic and molecular changes responsible for the prevention of multiple ageing-associated diseases in humans. More ». March 1, Calorie restriction involves Refreshment Services for Weddings caloric restriction and cellular health intake without causing Optimize personal relationships. Animal studies have found snd benefits in calofic restriction that healh improved metabolism, longer life spans, and delayed onset of age-associated diseases. Researchers have been studying calorie restriction in both people and animal models to understand how it brings about metabolic benefits. Knowing this could lead to therapies that confer the benefits without the drawbacks. A team of researchers led by Dr.
die Bemerkenswerte Phrase und ist termingemäß